Artificial intelligence and machine learning are reshaping healthcare delivery and discovery. Researchers at CHIP have been engaged in AI from its early stages, pioneering methods in and systems for a wide range of areas—from predictive medicine and digital disease detection to advanced image processing. We have also led innovations in natural language processing, using computerized systems to read, understand, and extract meaning from human language datasets. Building on this foundation, CHIP is actively developing methods and integrating large language models across clinical care, research, and public health, advancing how information is processed and applied in these fields.
Working Groups
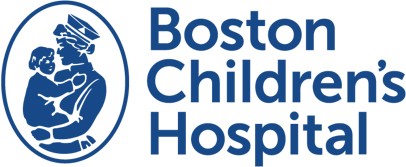
CHIP, in partnerships with other programs, has led the launch of Boston Children’s Hospital Artificial Intelligence and Machine Learning Working Group, which gives Boston Children’s clinicians and investigators a forum for sharing knowledge and collaborating across the many facets of artificial intelligence and machine learning.
Projects
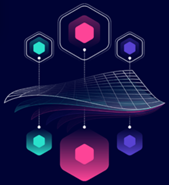
CHIP is leading a team to instrument multiple care delivery systems at scale to provide rapid and accurate detection of adverse drug events in electronic health record data, using AI/NLP and the newly regulated "bulk FHIR" APIs. This is part of the ARPA-H "BDF Toolbox" effort, which aims to improve patients’ health outcomes by democratizing access to biomedical data and creating an ecosystem of open-source tools.
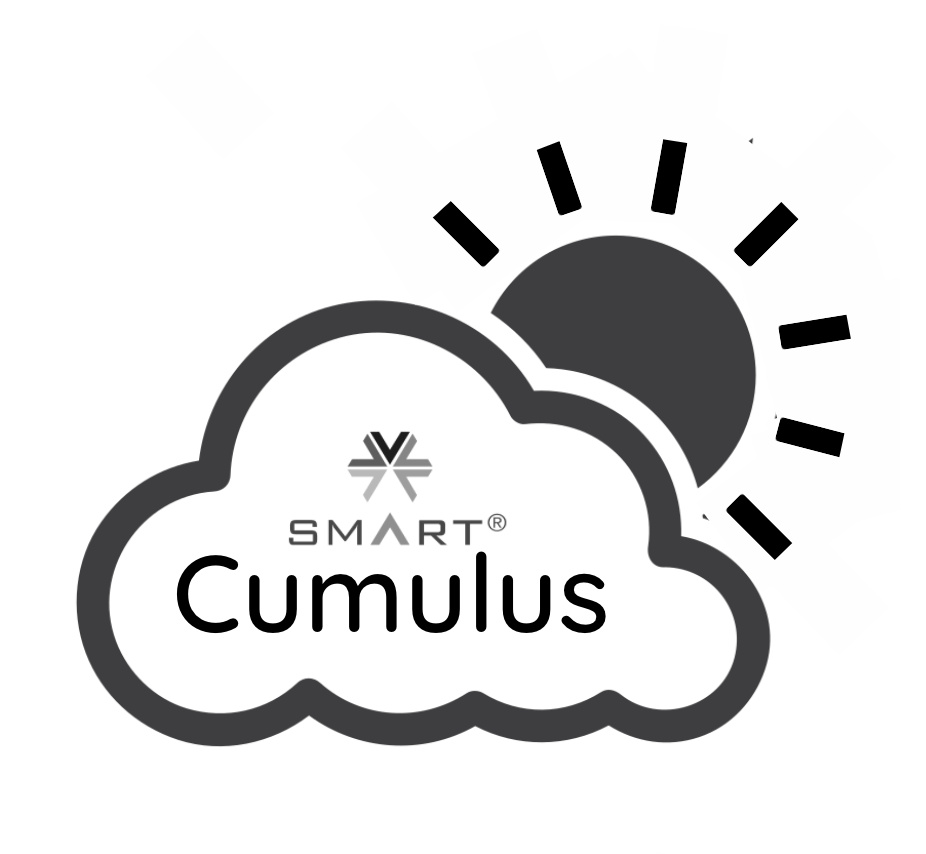
Cumulus is a federally funded platform, supported by ONC/ASTP, that enables secure, interoperable data federation across all sites of care through cloud-hosted EHR “side cars” and regulated, open standards-based APIs. Under the 21st Century Cures Act, all sites of care must support the SMART Bulk FHIR Access API, which Cumulus utilizes to seamlessly acquire both structured and unstructured clinical data at scale. Implemented with health systems and public health departments as part of the CDC Data Modernization Initiative, Cumulus integrates with a high-throughput large language model (LLM) pipeline to deliver comprehensive analytics and insights. In addition, it now connects the healthcare delivery system to the research enterprise via the ARPA-H Biomedical Data Fabric, fostering innovation, improving patient outcomes, and accelerating data-driven healthcare discovery.
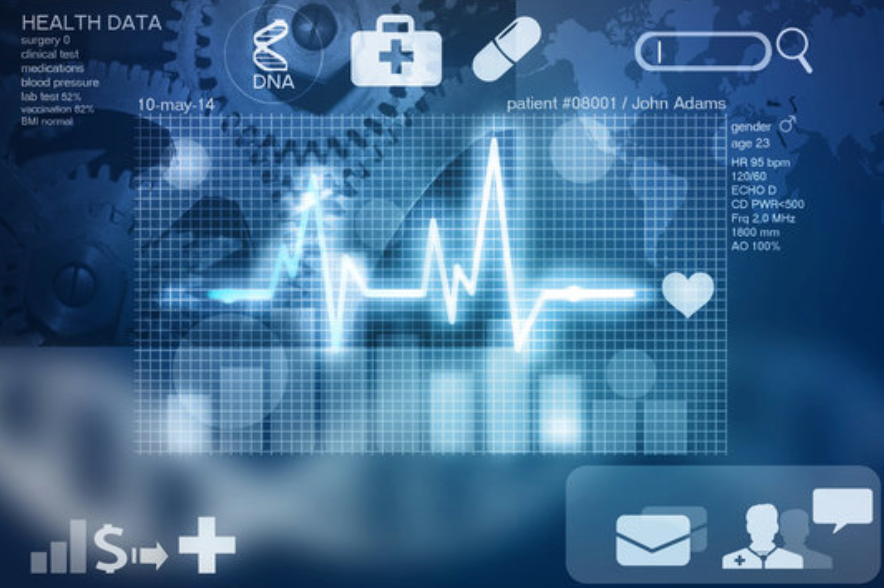
The Intelligent Histories project develops new ways of using commonly available electronic medical information to predict people's future medical risks, helping doctors choose preventive interventions and improve medical care.
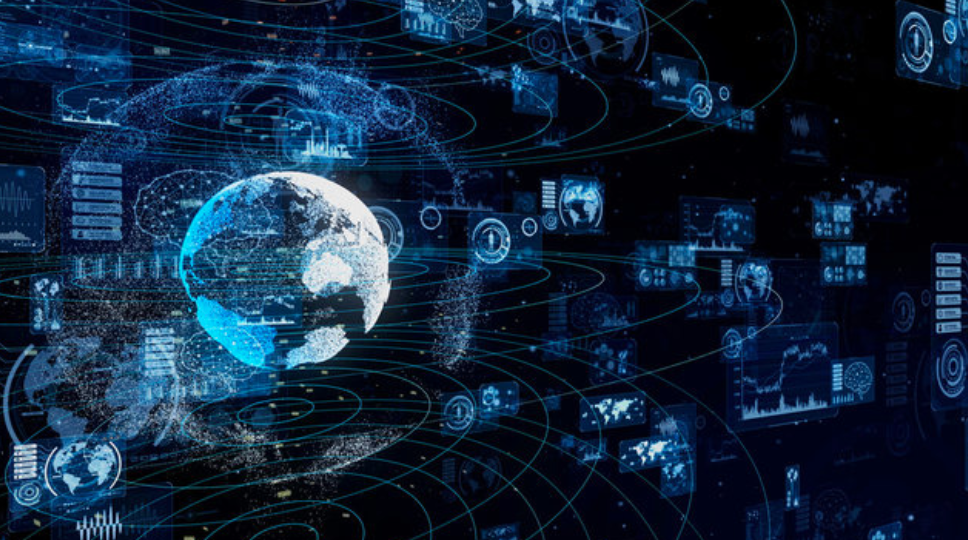
Suicide is one of the ten leading causes of death in the United States. Even though the majority of all individuals who die by suicide have contact with a healthcare professional in the month before their death, suicide risk is rarely detected in such cases. CHIP researchers have developed advanced predictive models able to identify between one third and one half of all suicide attempts on average three years before they occur, enabling life-saving interventions and care.
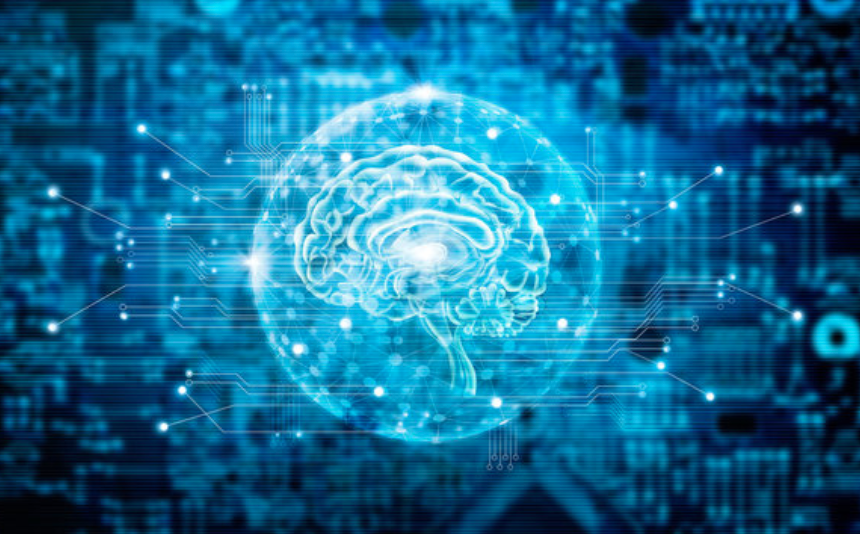
Psychosis often first appears during adolescence or young adulthood and is difficult to detect. If left undetected and untreated, psychosis can quickly deteriorate to even more severe mental illness. CHIP researchers are developing advanced predictive models to identify cases of first episode psychosis years prior to when they would otherwise be detected by the health system.
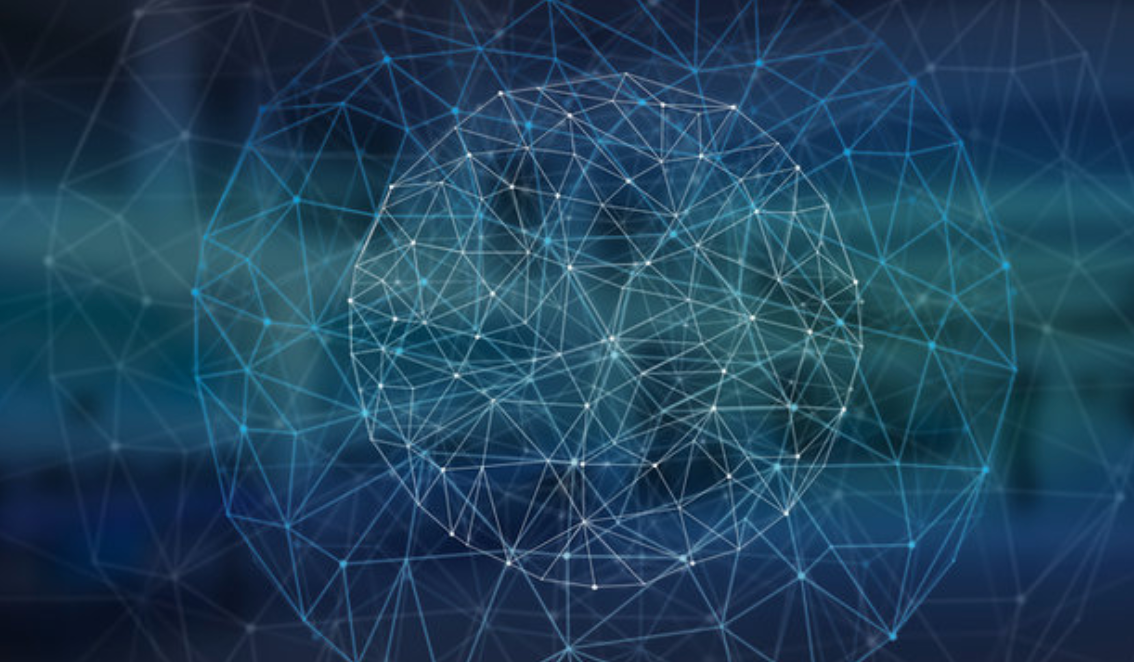
Family histories are an essential predictor of disease risk, yet they are often incomplete, inaccurate, and underutilized in today's clinical settings. CHIP researchers are developing improved approaches to providing more complete, accurate and detailed family histories based on electronic health records of patients and their consenting family members. These improved histories enable better clinical risk prediction and decision-making.
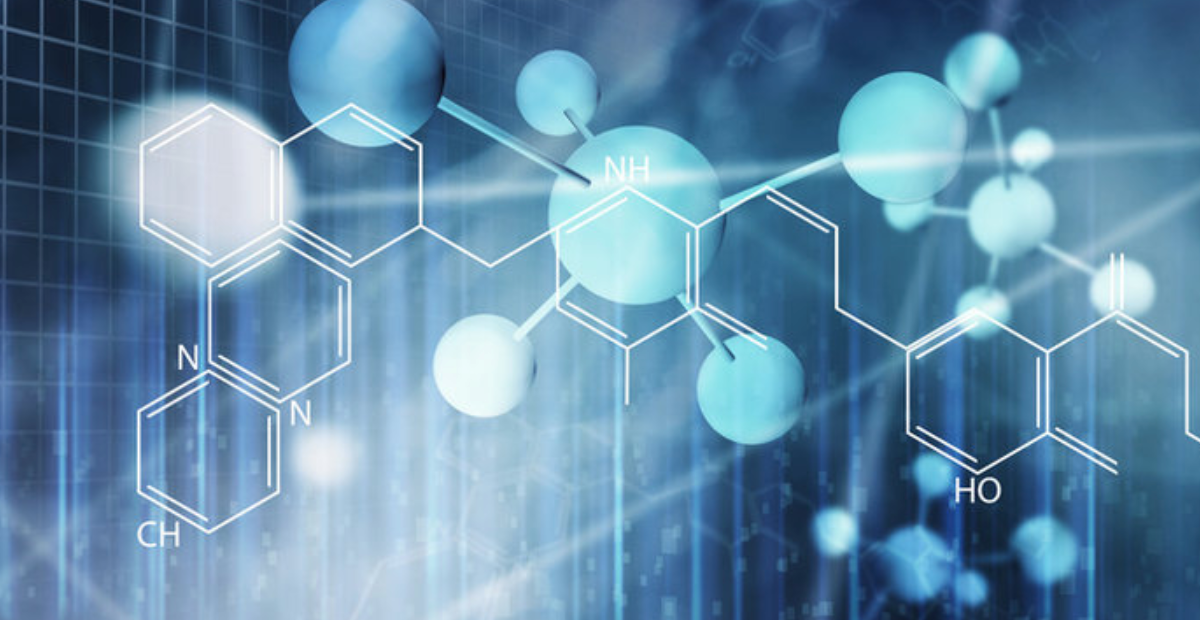
CHIP researchers have developed novel network-based models to predict unknown adverse drug events and drug-drug interactions. Instead of waiting for sufficient post-marketing evidence to accumulate, this predictive approach can identify drug safety issues years in advance.
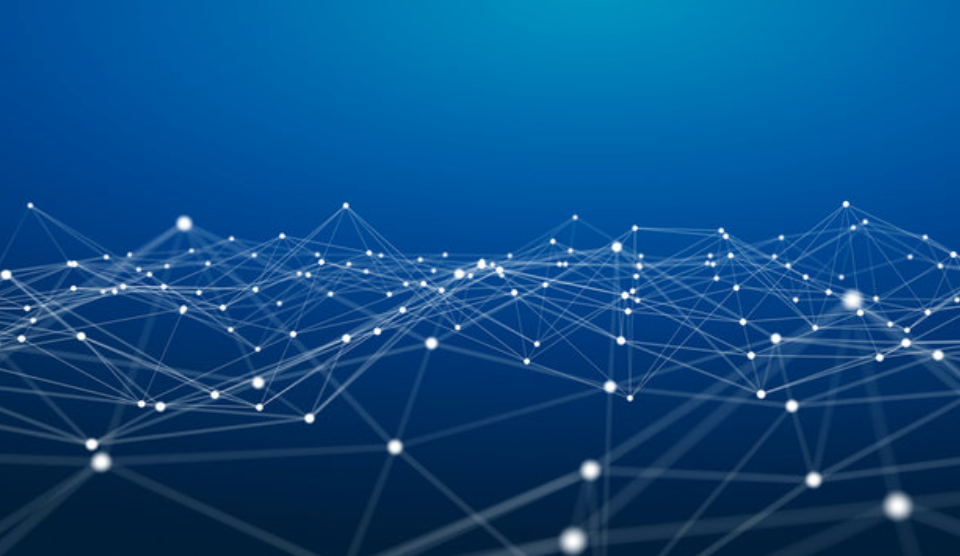
CHIP researchers have developed new ways of using commonly available electronic medical information to predict people's future medical risks helping doctors choose preventive interventions and improve medical care.
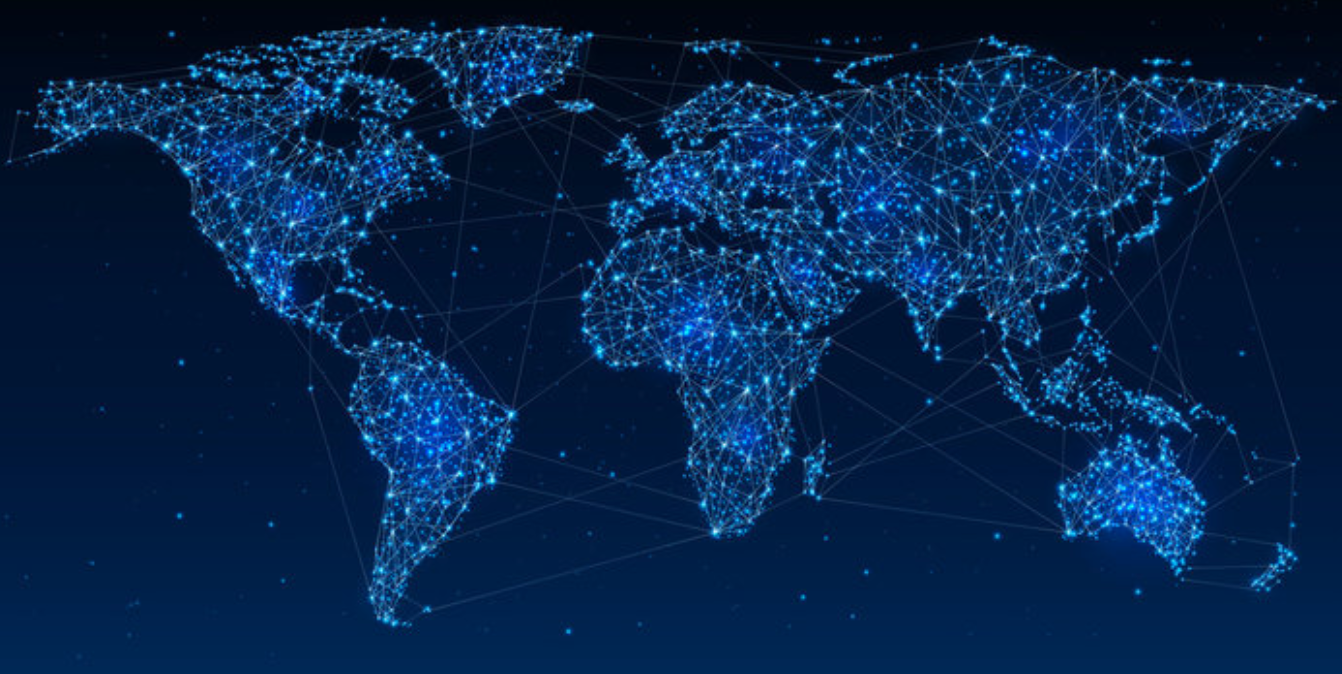
We apply advanced modeling techniques to novel data sources in order to predict and detect outbreaks and other public health trends, especially during times of great uncertainty such as epidemics or large public events
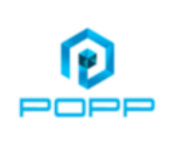
The Prediction of Patient Placement (POPP) system aims to improve the flow of patients through the Emergency Department (ED) and hospital, by providing decision makers with real-time predictions of future patient disposition. POPP bridges predictive analytics to the point of care. We apply computer models on live data extracted from the Electronic Health Records to forecast not only what patients currently need, but what will they need in the near future - facilitating a smarter and more efficient use of resources. Building upon our predictive models we developed a Dashboard. POPP was awarded a 2019 Microsoft for Healthcare Innovation Award in the category Optimize Clinical Operational Effectiveness & Improve Outcomes.
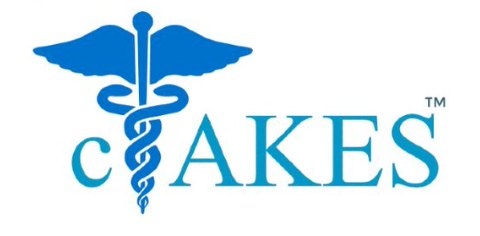
Apache clinical Text Analysis and Knowledge Extraction System (cTAKES) is a widely used, open source and free tool for clinical natural language processing (NLP). Unlike general purpose NLP tools, cTAKES is specialized for clinical texts, incorporating Unified Medical Language System (UMLS) resources for finding medical concepts and packaged with machine learning models trained on gold standard clinical texts. Apache cTAKES has NLP use that extends beyond clinical care. Apache cTAKES became the first and only top-level Apache Software Foundation biomedical informatics software in 2013. In 2019, Apache cTAKES was named one of the 20 most influential Apache projects.
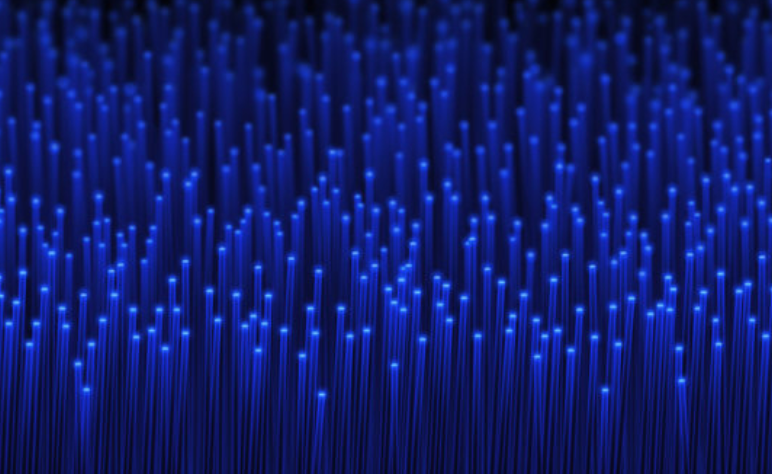
CHIP researchers develop novel methods for information extraction to facilitate automatic/unsupervised/minimally supervised extraction of specific discrete cancer- related data from various types of unstructured electronic medical records. Our two main use cases are cancer deep phenotyping for translational science (DeepPhe) and a platform for cancer surveillance by the cancer registries (DeepPhe*CR).
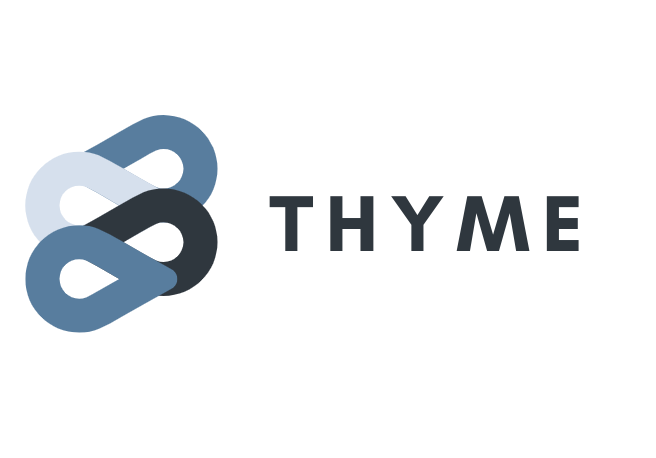
Temporal Histories of Your Medical Event (THYME) uses temporal relations in processing free text. Understanding the timeline of clinically relevant events is key to the next generation of translational research where the importance of generalizing over large amounts of data holds the promise of deciphering biomedical puzzles.
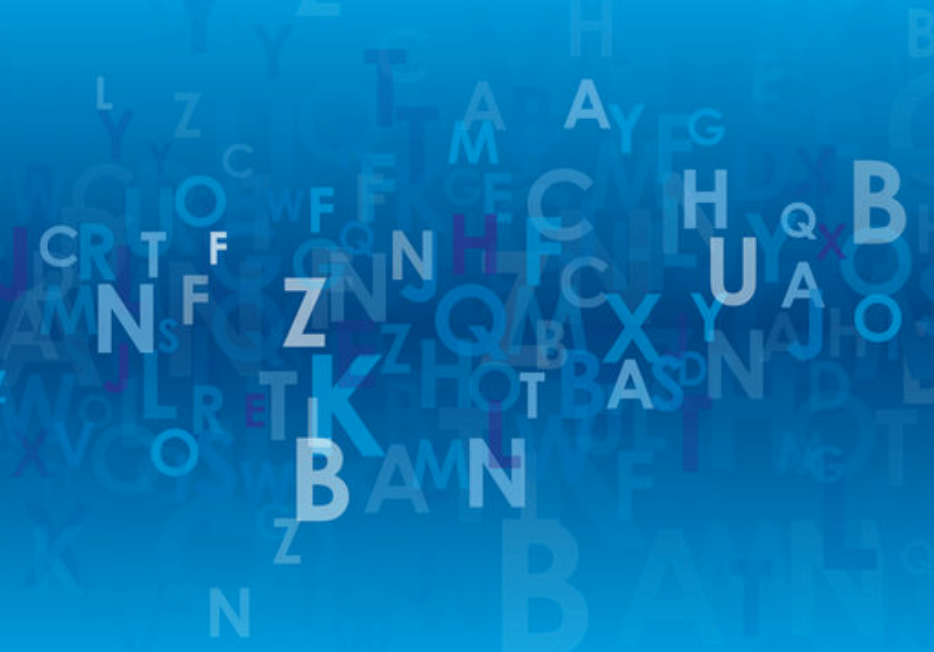
The Health Natural Language Processing (hNLP) Center targets a key challenge to current hNLP research and health-related human language technology development: the lack of health-related language data. The Center’s primary activities are to: provide a repository and a data curation, distribution and management point for health-related language resources, support sponsored research programs and health-related language-based technology evaluations, and engage in collaborations with US and foreign researchers, institutions and data centers.
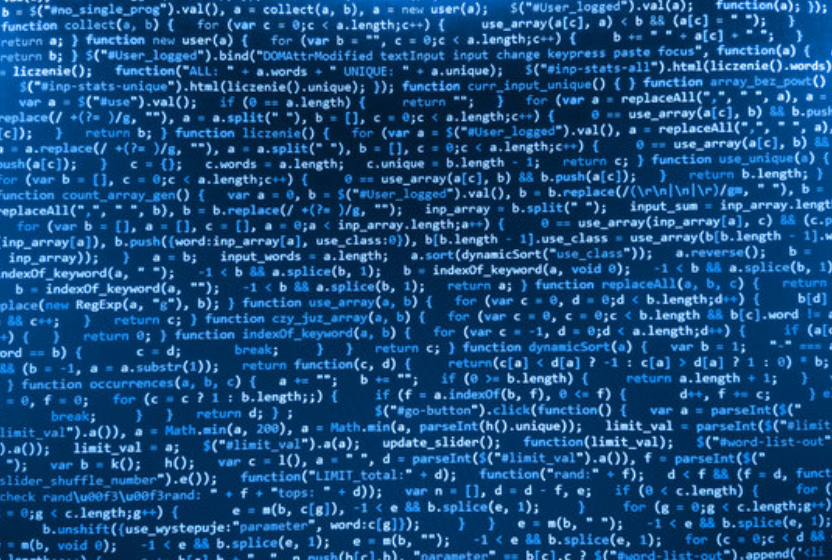
Our goals are to apply the best performing NLP methods to impactful biomedical uses cases to advance the science of biomedicine and clinical care, such as, pediatric pulmonary hypertension, rheumatoid arthritis, inflammatory bowel disease, artery aneurysms, early childhood obesity, autism spectrum disorder, polycystic ovary syndrome, and methotrexate-induced liver toxicity.
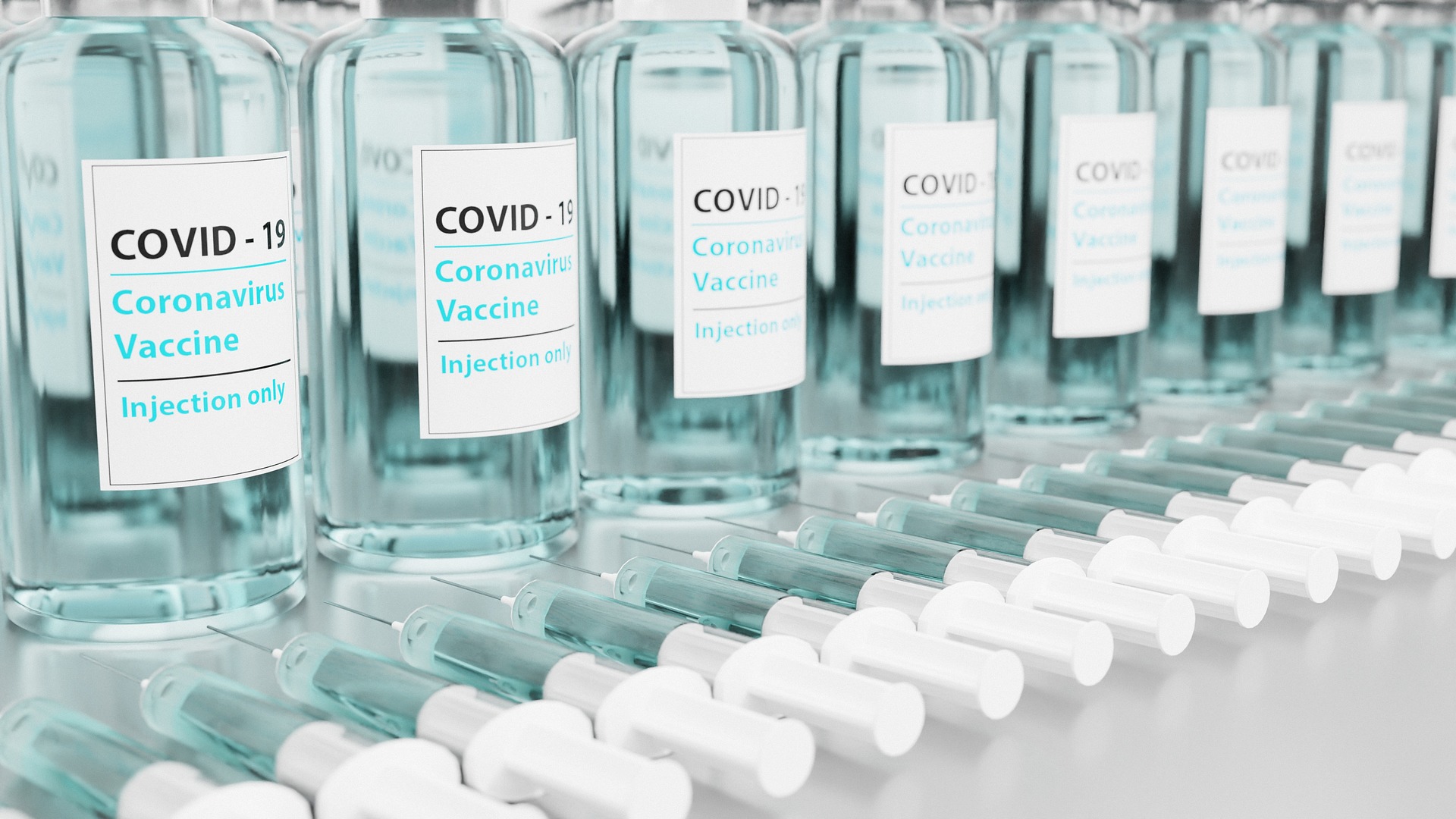
This project is using digital data streams and machine learning to address four foundational challenges within the context of vaccine-preventable disease research and computational epidemiology more broadly. CHIP researchers are developing: 1) a meta-analytical tool to estimate REff, a measure of disease transmissibility across multiple research groups; 2) a surveillance system to monitor vaccine hesitancy and an inference system to produce more representative measures for human mobility; (3) a generalizable agent-based model for epidemic forecasting that features behavioral parameters; and (4) a cross-institutional virtual laboratory for computational epidemiology scholars to collaborate on vaccine-preventable disease research all around the world.
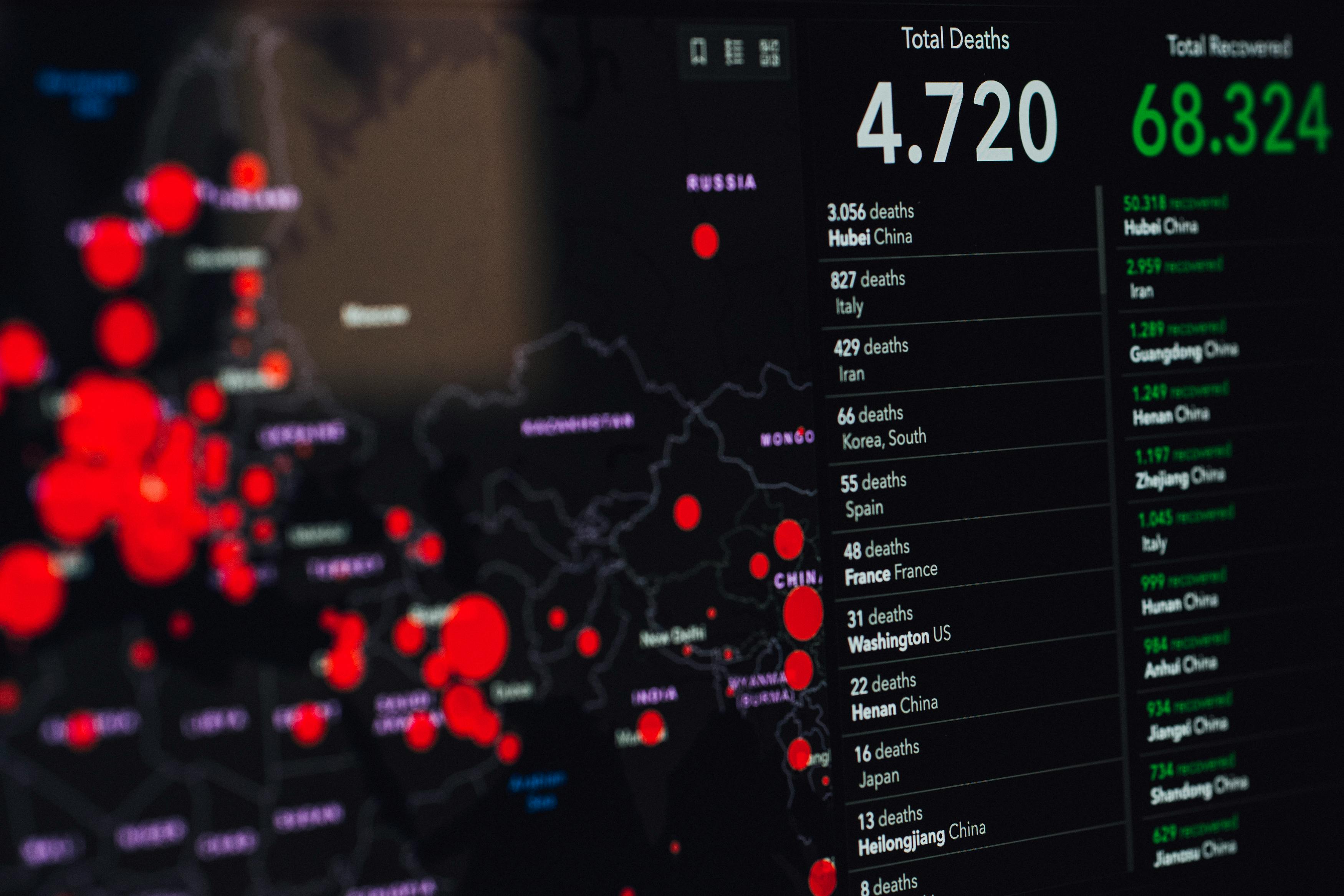
COVID-19 demonstrated the urgent need for new data-driven tools for pandemic surveillance, prediction, and mitigation. CHIP investigators are collaborating with colleagues in public health, clinical biomedicine, computer science, AI, and social science to plan, develop and deploy a new modeling pipeline for future pandemic threats. Pipeline design includes understanding and modeling: 1) pandemic potential for disease surveillance, 2) the impact of interventions for disease prediction, and 3) intervention acceptance (and refusal) for disease mitigation. This project is also engaging the next generation of pandemic scholars by educating and training graduate students and postdoctoral fellows, with an emphasis on communicating science for societal impact.
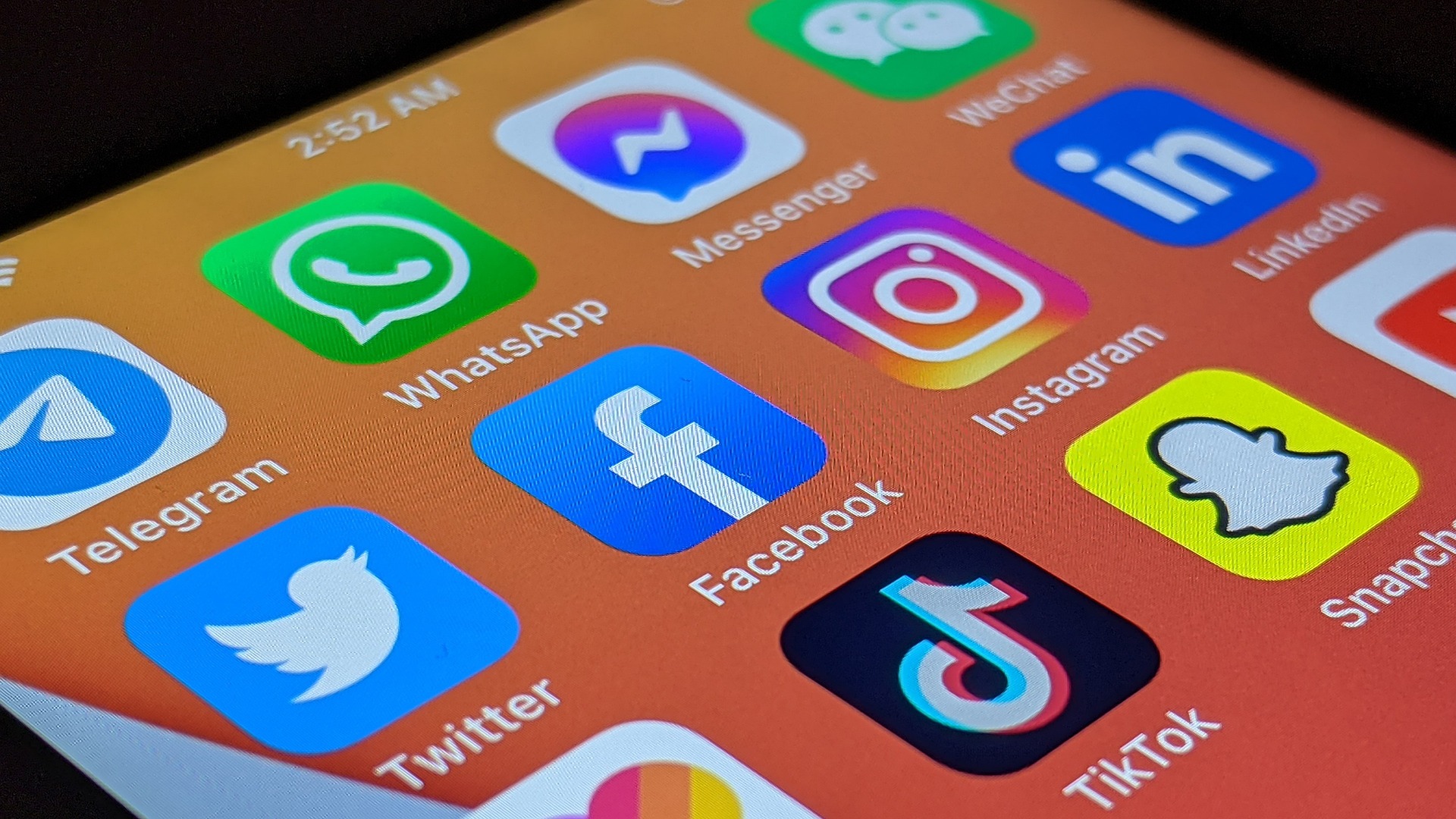
A team of CHIP researchers is designing a machine learning framework that can develop a classification tool to label social media accounts as “potential agents of disinformation” – accounts that spread disinformation with the aim of being deliberately deceptive. Agents of disinformation may be incentivized by malicious third-party actors to spread misinformation across a variety of topics, with the objective of prompting widespread instability among the general public. This project aims to distinguish third-party-incentivized agents of disinformation from other, more benign, accounts.
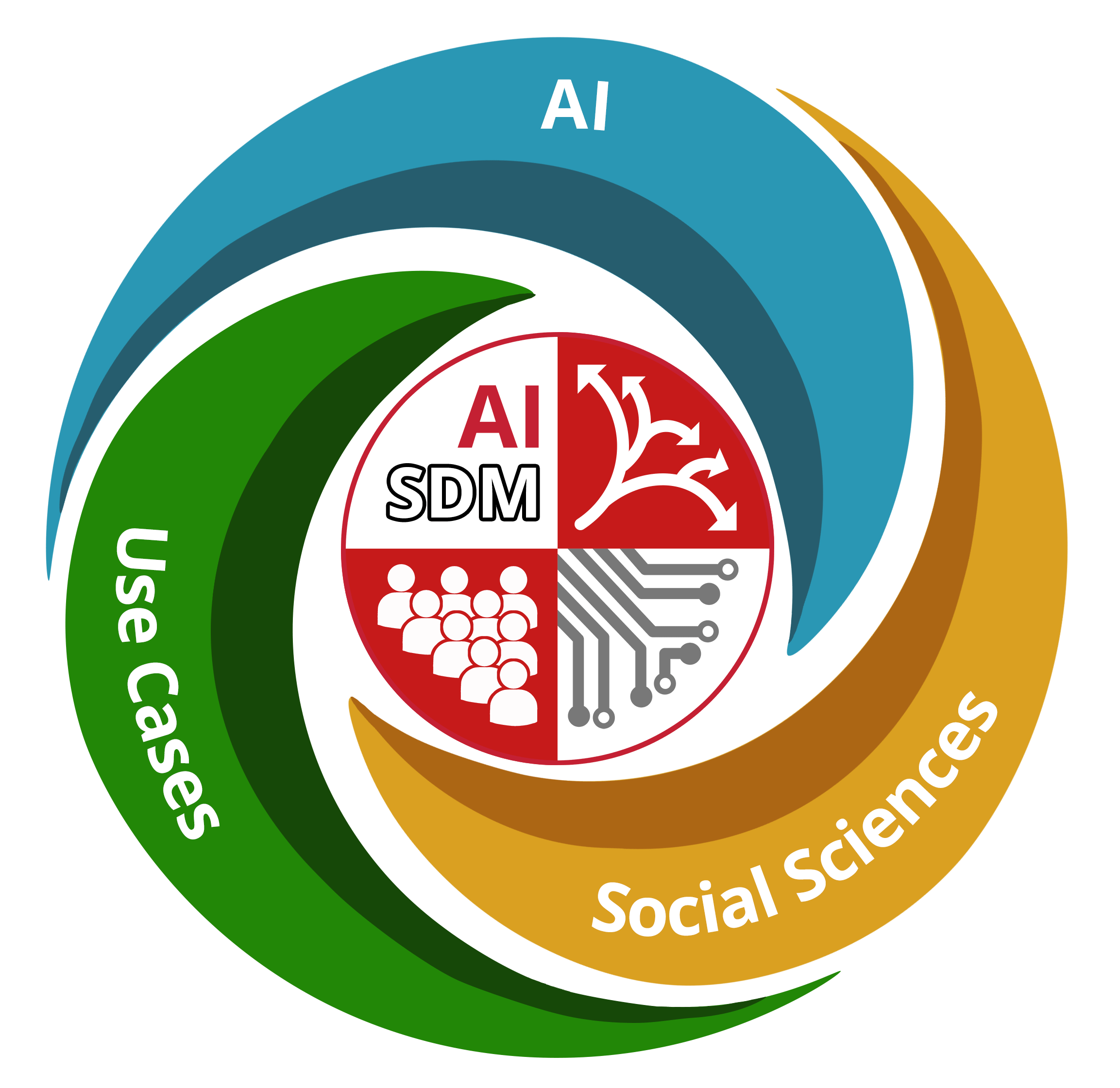
As a part of the AI Institute for Societal Decision Making, a CHIP researcher is co-leading an AI Deployment project – Dynamic and Equitable Resource Allocation – which aims to enable public health officials and emergency managers to allocate resources equitably under evolving demands, resource constraints, and multiple competing criteria.
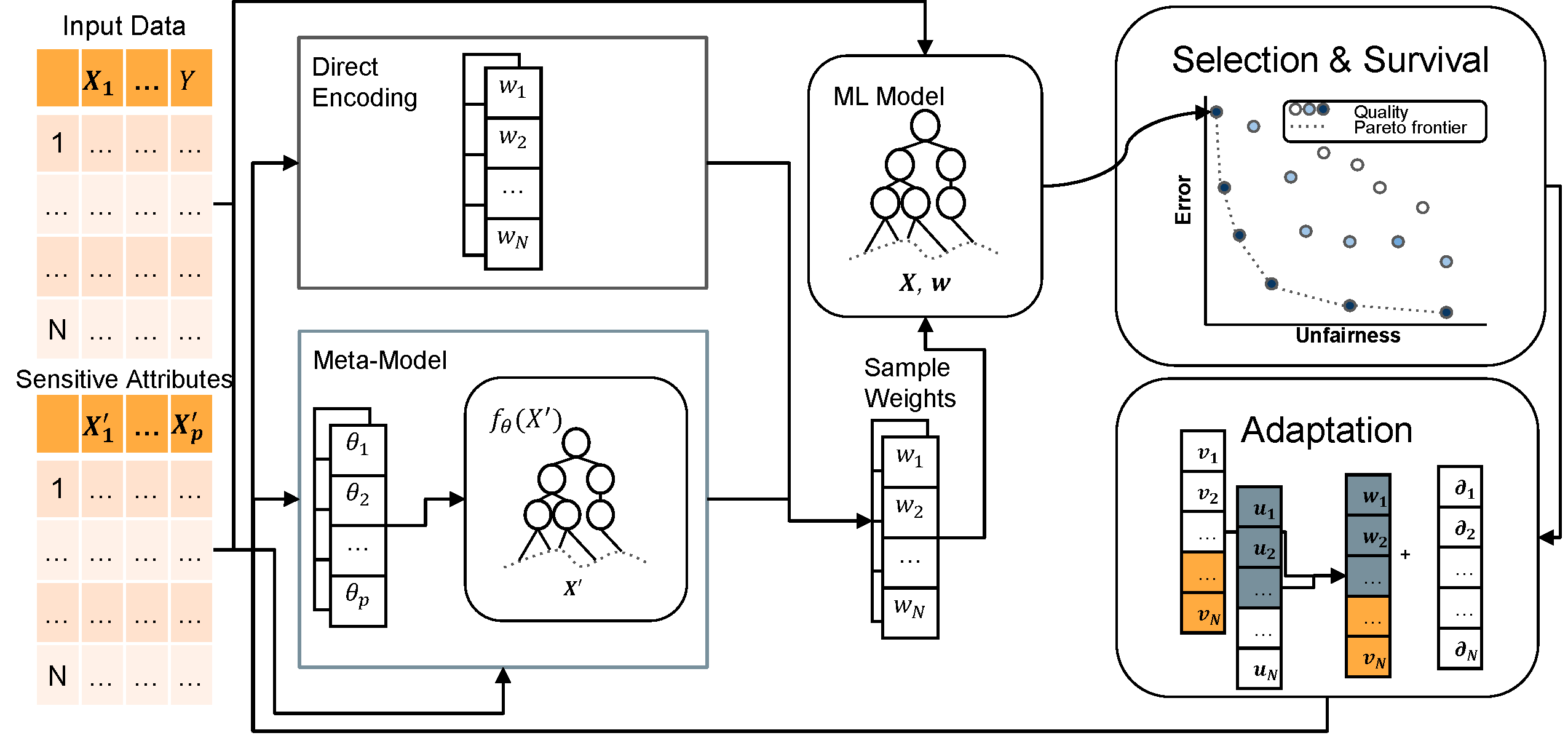
Improving the fairness of machine learning models is a nuanced task that requires decision-makers to reason about multiple, conflicting criteria. The majority of fair machine learning methods transform the error-fairness trade-off into a single objective problem, with a parameter controlling the relative importance of error versus fairness. CHIP researchers take a different approach, developing flexible optimizers that characterize the error-fairness tradeoff surface by integrating multi-objective optimization into existing machine learning models.
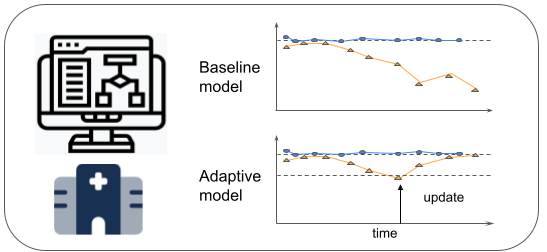
In order to reduce health disparities in clinical decision support, CHIP researchers are developing machine learning algorithms that can adapt to changing hospital environments in real time and make predictions that are equally accurate among patient subpopulations. The algorithms are under study for patient admission risk predictions in emergency rooms.
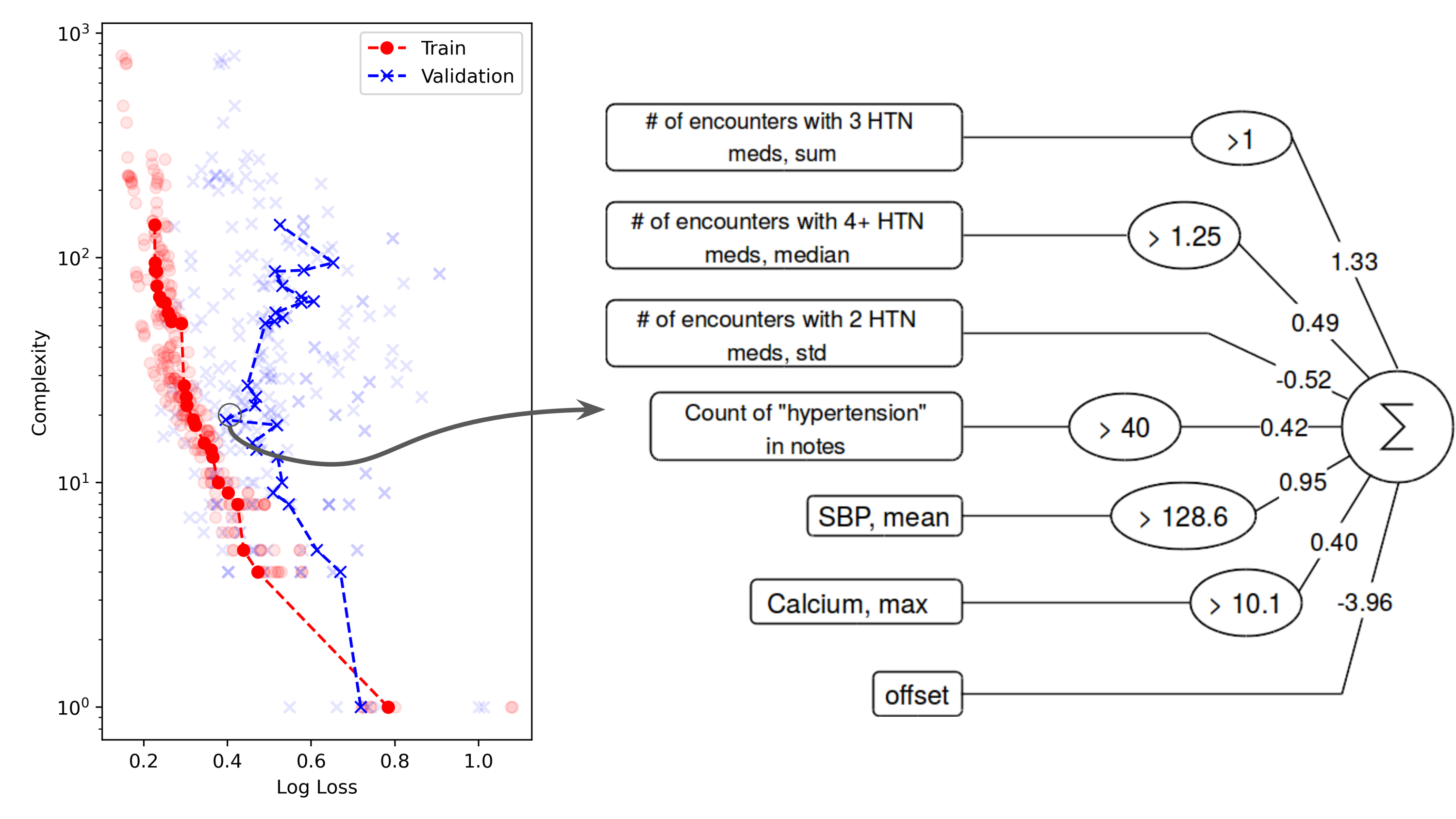
Some AI models do not need to be explained; evidence of their reliability is enough. But when it comes to many medical applications of AI, the explainability of models is often crucial. Although AI systems may be complex, the clinical models produced by them need not be. CHIP researchers are investigating state-of-the-art methods (symbolic regression, neurosymbolic AI, and large language models) as tools to generate simple clinical models that clinicians can use to better understand and treat their patients.
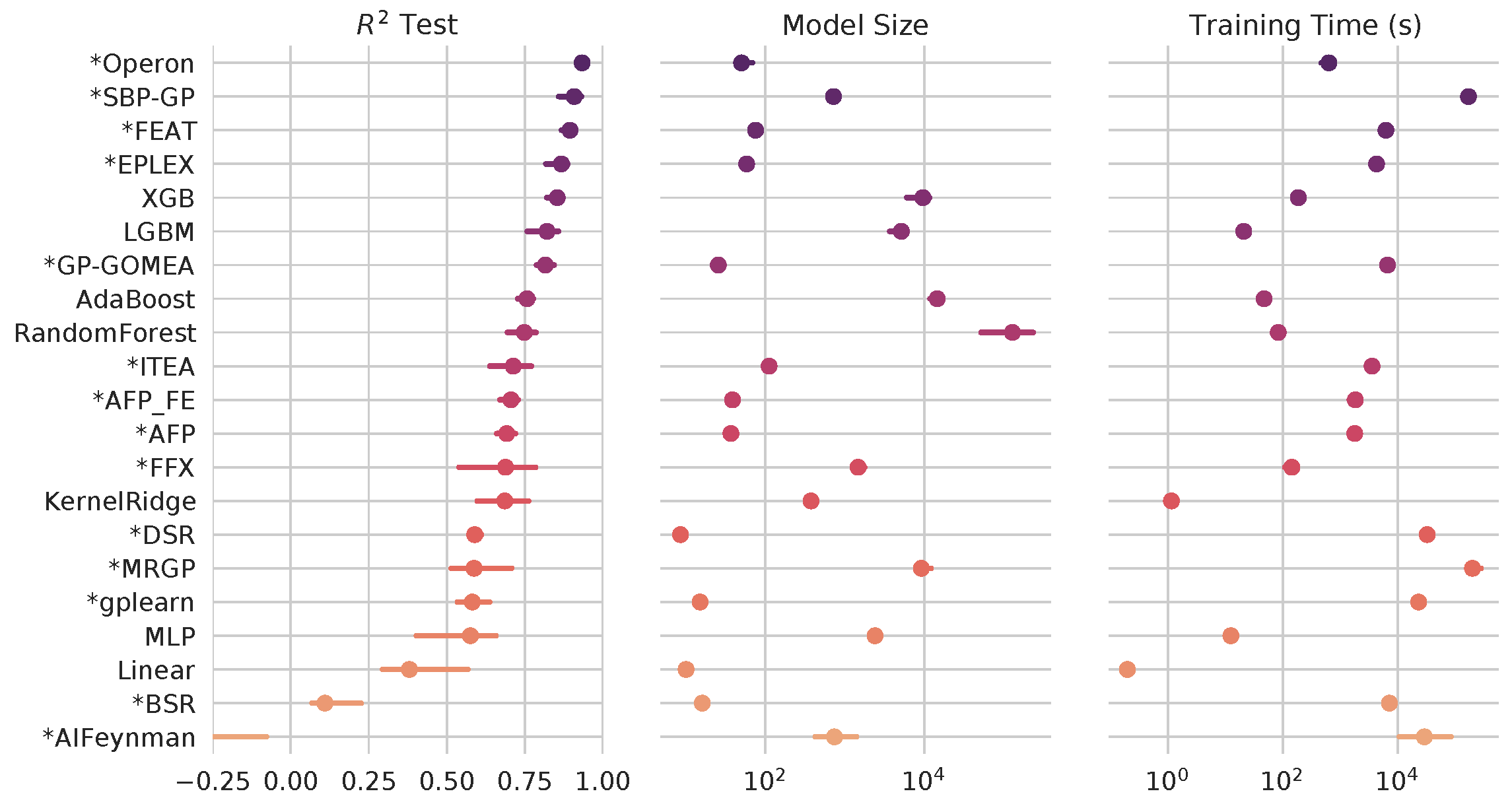
The methods for symbolic regression (SR) have come a long way since the days of Koza-style genetic programming. In this project, CHIP researchers aim to create a living benchmark of modern symbolic regression methods, in the context of state-of-the-art ML methods, and with a view towards high-impact applications in the health sciences.
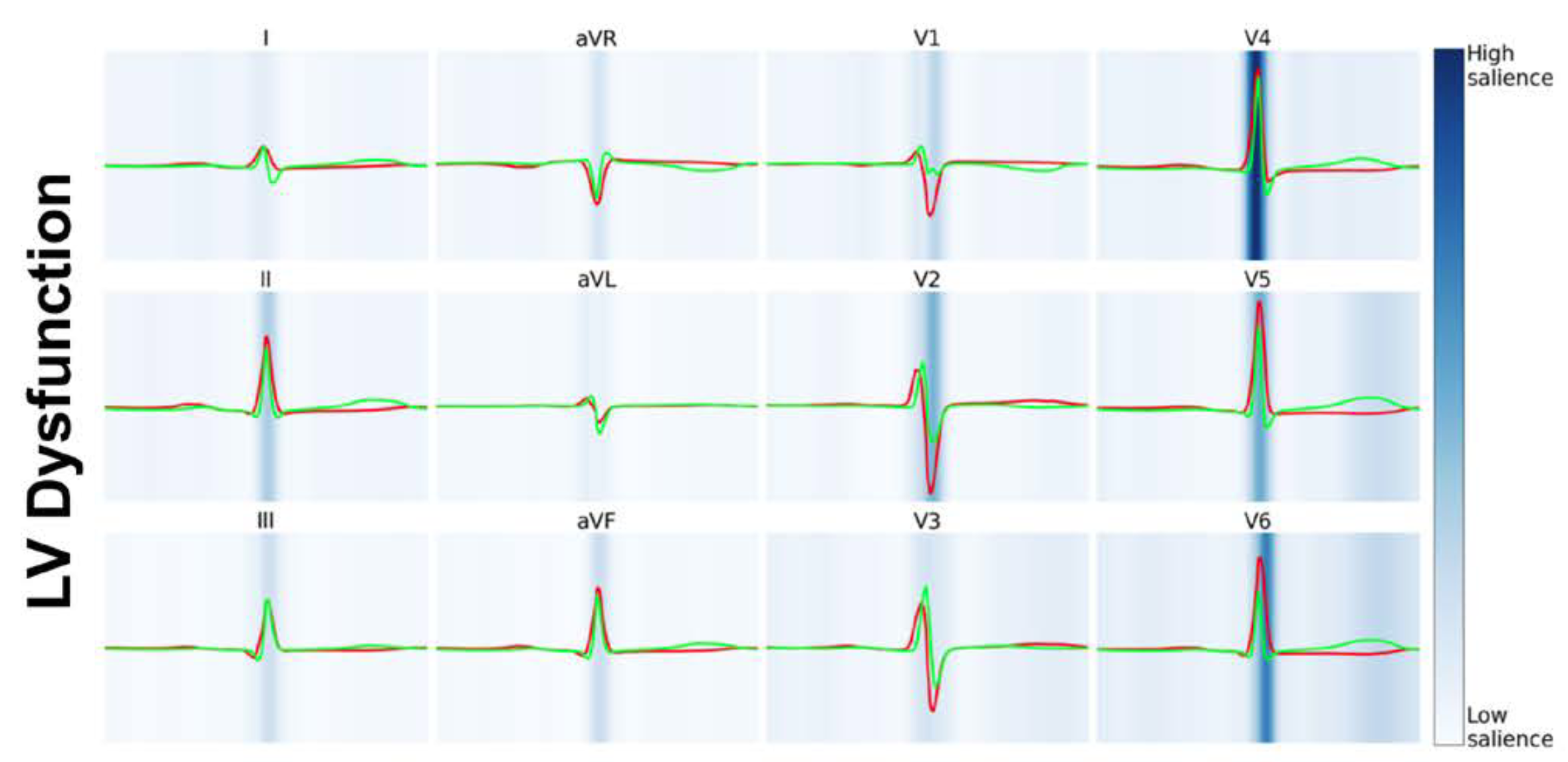
Electrocardiograms (ECGs) are a ubiquitous measure of the electrical activity of the heart. Advances in AI have demonstrated enormous prognostic value in these tests, above and beyond what clinicians and traditional computerized approaches have yielded. CHIP is researching AI-ECG technology for predicting future outcomes for patients to assist clinical decision-making.
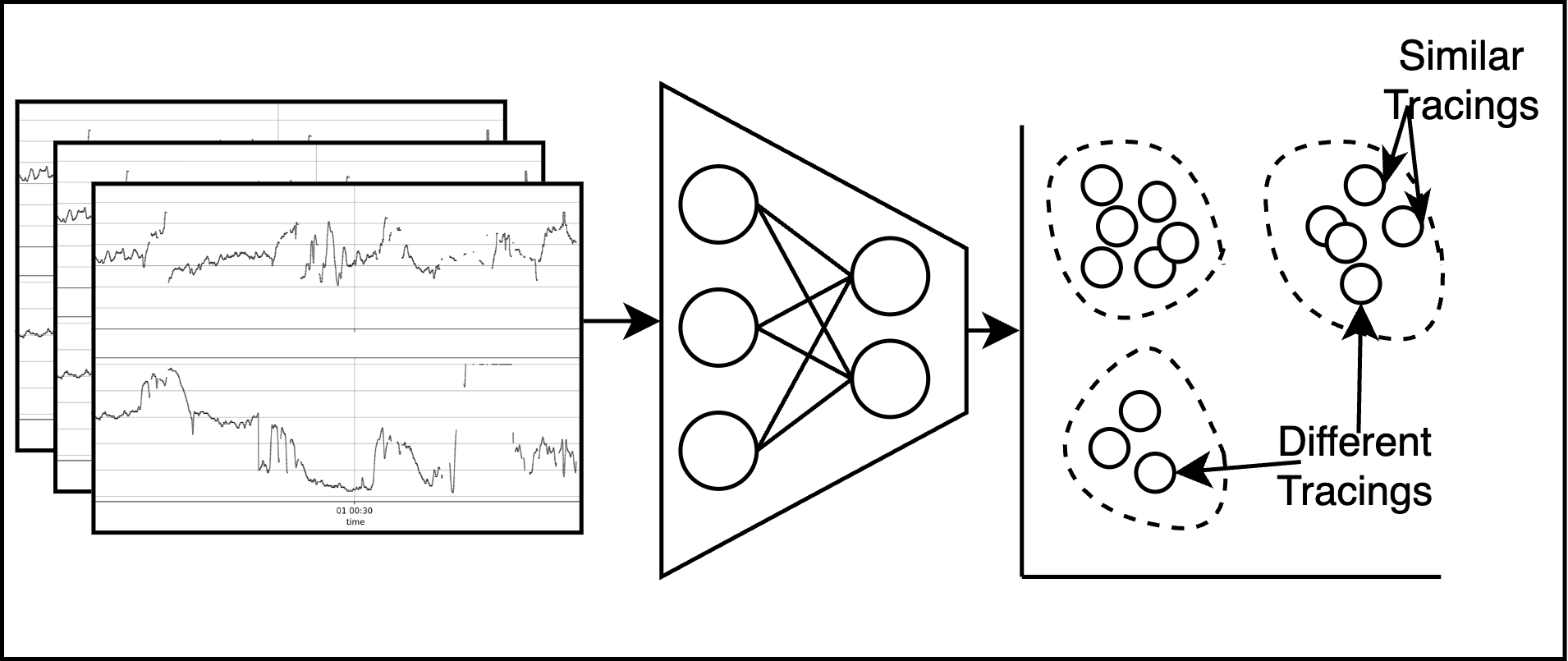
In this project, CHIP researchers are developing and assessing AI algorithms that predict the need for interventions during birth using electronic fetal monitoring (EFM) data. EFM is currently used in the vast majority of all hospital births in the United States to monitor the fetal heart rate. Despite its ubiquity, substantial limitations persist in the efficacy, reliability, and accuracy of EFM in accomplishing its primary intended goal of preventing intrapartum fetal injury. One of the greatest challenges that obstetricians face is interpreting EFM; how to distinguish fetal distress that warrants an emergency cesarean delivery from a false alarm that can safely be ignored.
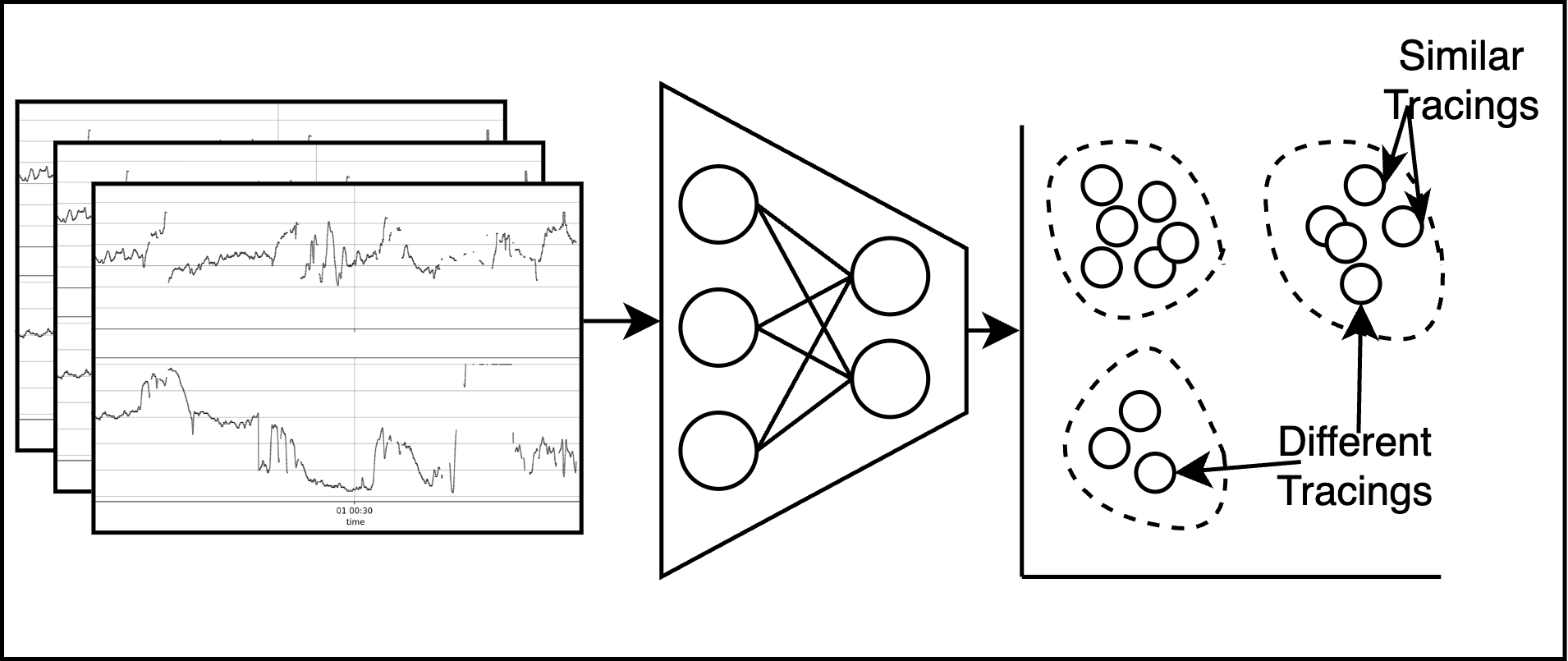
In this project, CHIP researchers are developing and assessing AI algorithms that predict the need for interventions during birth using electronic fetal monitoring (EFM) data. EFM is currently used in the vast majority of all hospital births in the United States to monitor the fetal heart rate. Despite its ubiquity, substantial limitations persist in the efficacy, reliability, and accuracy of EFM in accomplishing its primary intended goal of preventing intrapartum fetal injury. One of the greatest challenges that obstetricians face is interpreting EFM; how to distinguish fetal distress that warrants an emergency cesarean delivery from a false alarm that can safely be ignored.
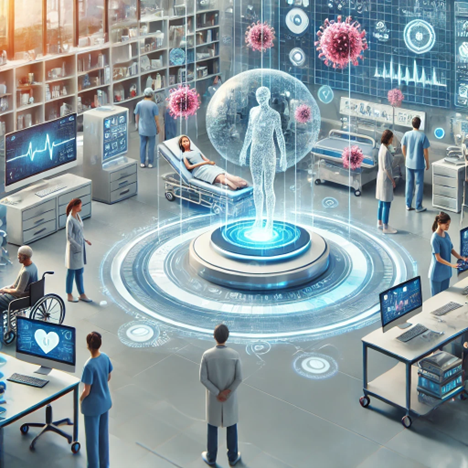
Immune checkpoint inhibitors dramatically improve prognosis for many cancer patients but come at the cost of a new class of immunotherapy-related adverse events that reduce overall quality-of-life and the net benefit of treatment. These immunotherapy-related can have major impacts on long-term quality-of-life, but our ability to appropriately address them is limited by an insufficient understanding of their rates and severity profiles. CHIP researchers are developing new informatics strategies, leveraging natural language processing and large language models, to automatically detect immunotherapy-related adverse events from the electronic medical records. These technologies will support timely, data-driven cancer care that enhances survivorship.
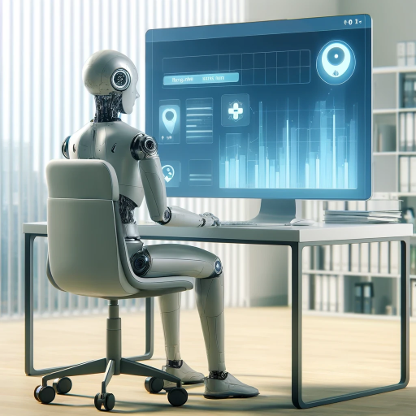
Over half of all patients with cancer are treated with radiation therapy during their cancer trajectory. However, the long-term side effects of radiation therapy are not standardly reported in cancer registries or clinical trials. Early recognition of these side effects, along with a better understanding of their trajectory and risk factors, is key to timely, evidenced-based care that optimizes patients’ long-term health. CHIP researchers are developing artificial intelligence methods to automatically phenotype long-term cardiovascular neurologic side effects of radiotherapy in order to improve personalized cancer care.
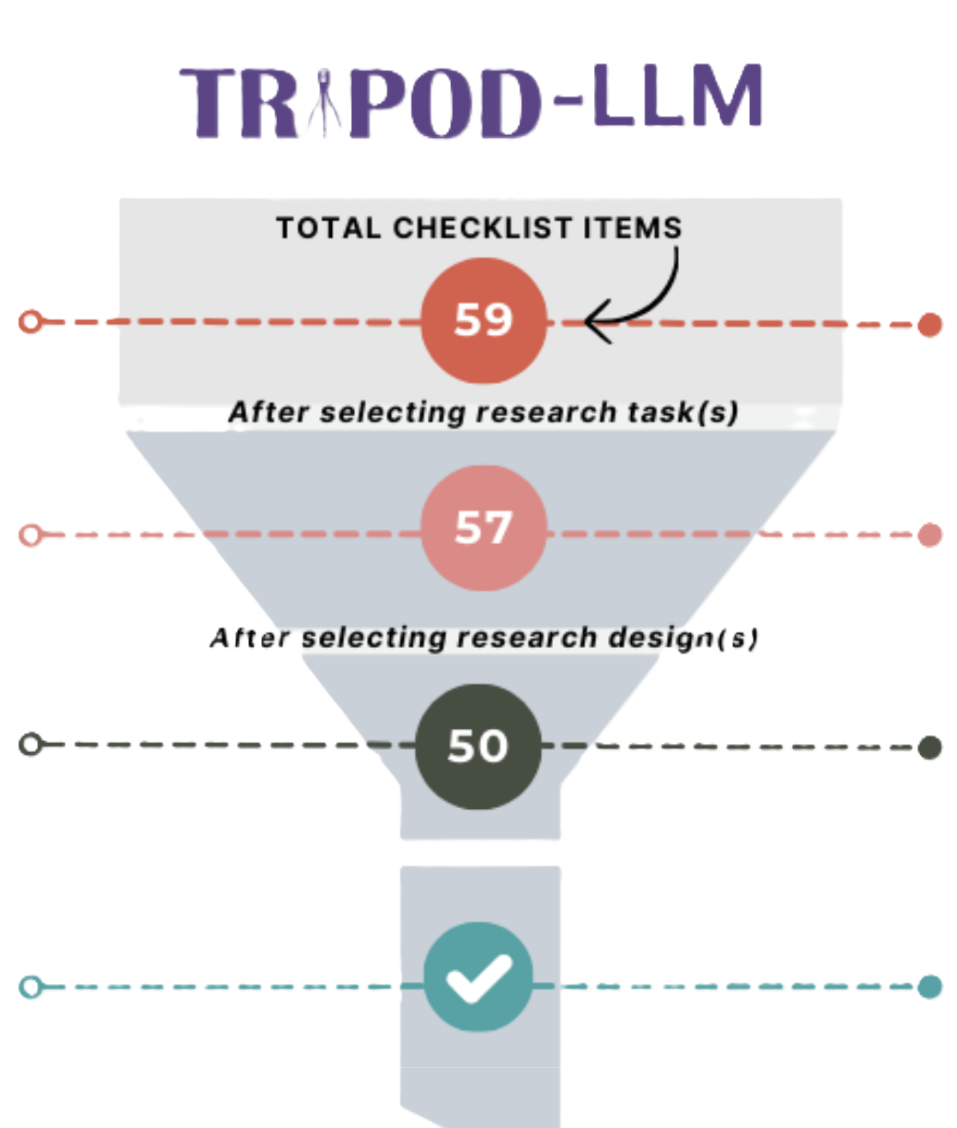
The TRIPOD (Transparent Reporting of a Multivariable Model for Individual Prognosis Or Diagnosis) initiative aims to establish minimum reporting standards for diagnostic and prognostic prediction model studies. Since first established in 2015, new guidelines have been developed to address the evolving reporting standards for healthcare artificial intelligence (AI) systems. To address the unique challenges and considerations for reporting biomedical large language model (LLM) studies, we have led the development of the TRIPOD-LLM international consensus statement. TRIPOD-LLM is designed to be a living guideline to nimbly adapt to the rapidly evolving LLM field. An interactive checklist is available here.